Understanding AI and Its Significance
Artificial Intelligence (AI) is quickly becoming a popular topic within and outside of the scientific community. AI technology includes machine learning (ML), deep learning (DL), and AI itself. These can be thought of as a series of AI systems from largest to smallest, each encompassing the next IBM.
Examining the Growth of AI
The global machine learning market was valued at $8 billion in 2021 and is anticipated to reach USD 117 billion by 2027, growing at a 39 percent CAGR EES Corporation. This explosive growth in AI and its subsets points to their increasing importance and potential for future advancements.
The Continuous Development of AI
AI’s growth rate is not just limited to its economic value. The advancements in AI technology and its subset fields also mark a pace of development and promise significant improvements in various sectors.
Exploring Machine Learning
Machine Learning (ML), a vital subset of AI, focuses on the development of computer programs that can access data and use it to learn for themselves. By giving machines the ability to learn, we increase their efficiency and have seen exceptional growth in this area.
Focusing on Deep Learning
Deep learning, an advanced division of machine learning, uses artificial neural networks with several abstraction layers to produce state-of-the-art accuracy in tasks real-time object detection, speech recognition, image classification and more Springer.
The Role of Neural Networks
In AI, a neural network takes its inspiration from biological neurons and the network they form. Artificial Neural Networks (ANN) are critical to the workings of AI, especially in fields such as deep learning and machine learning Springer.
AI: Painting the Future
Given the growth rates projected for the AI industry, experts believe that AI and its subset fields could revolutionize numerous sectors, including healthcare, education, finance, and more. These advancements are eagerly anticipated, with new technologies in AI promising a future of enhanced efficiency and revolutionary changes.
Development projections and potential challenges
While we can expect consistent development in AI, potential challenges need to be considered. Key among these is the ethical implications of AI development, protection of data privacy and the potential for job disruptions in certain sectors.
Importance of Embracing AI Growth
As we continue into an increasingly digital era, embracing AI and its growth becomes even more crucial. AI has the potential to revolutionize how we interact with technology, making our lives easier and more efficient.
What does the projected growth of AI mean for the future?
What potential challenges are related to the growth of AI?
To quote renowned physicist Stephen Hawking: “Artificial Intelligence could be the biggest event in the history of our civilization. Or the worst. We just don’t know. So we cannot know if we will be infinitely helped by AI, or ignored by it and side-lined, or conceivably destroyed by it.”. This highlights the importance of the careful and considered development of AI technology, given its potential impact.
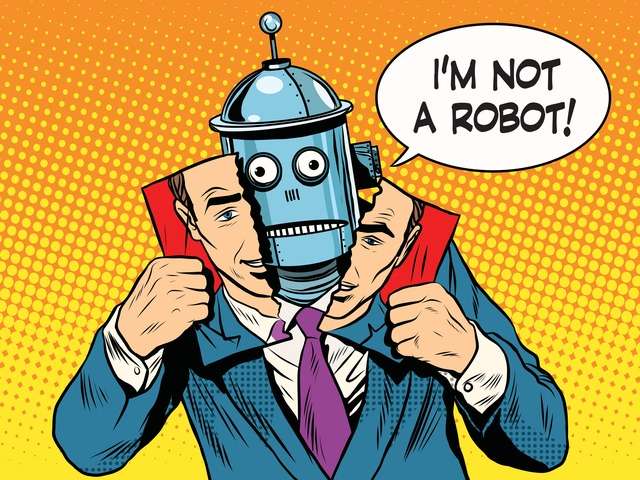
In the realm of artificial intelligence (AI), machine learning, and deep learning, crafting algorithms for neural networks is a necessary and complex process. These algorithms form the backbone of various AI-enabled systems that we see today – from voice assistants and recommendation systems to autonomous driving and climate modeling. This article describes the art of crafting effective neural networks algorithms.
Understanding Neural Networks
Neural networks are computational systems inspired by the biological neural networks constituting animal brains. These systems learn to perform tasks by analyzing examples, usually without task-specific programming. For instance, in image recognition, a neural network might learn to identify images containing cats by analyzing examples of cat images. The underlying neural networks algorithms perform this discernment.
The Art of Building Algorithms
There’s a science and an art to creating algorithms for neural networks. It requires a deep understanding of the problem at hand, the available data, and how different algorithmic approaches might solve the problem. One must understand AI modeling, data science, and computational intelligence create a well-performing and efficiently operating neural network. Engineers also need to consider the ethical implications of their algorithms, ensuring they operate fairly and without bias. Learn more about the role of artificial neural network and machine learning here.
Data: The Fuel of Neural Networks
The role of data in training, testing, and validating neural networks is fundamental. High-quality, diverse, and balanced data sets inform the crafting of effective neural network algorithms. It’s essential for engineers to know how to preprocess and clean data, handle missing data, and deal with potential outliers that may skew the results of the network.
The Aim for Simplicity and Efficiency
While solving complex problems often require complex models, one of the tenets of algorithm development is to strive for simplicity while achieving desired levels of performance or accuracy. Crafting neural network algorithms require achieving a fine balance between model complexity and efficiency. Overly complicated models may lead to overfitting, while overly simple models might fail to capture crucial patterns in the data.
Never-ending Process: Keep Training and Improving the Model
Developing highly efficient neural networks is a never-ending process. Even after deployment, these systems require constant monitoring and fine-tuning to maintain their performance and adapt to any changes in the input data.
Interpretable Machine Learning
Although the goal of a neural network is to make accurate predictions, it’s also important that these models be interpretable. This means that the model’s decision-making process should be understandable to its users, fostering trust and acceptance.
Ethical considerations in AI
Crafting neural network algorithms is not only about technological prowess. It also involves deep ethical considerations. For instance, engineers need to ensure their algorithms do not perpetuate harmful biases or have unintended consequences.
What are the key considerations in crafting neural networks algorithms?
Why is data important in building neural network algorithms?
As once stated by Andrew Ng, co-founder of Coursera and a former leader of Google Brain and Baidu’s AI Division, “Just as electricity transformed almost every industry 100 years ago, today I actually have a hard time thinking of an industry that I don’t think AI will transform in the next several years.”
According to McKinsey, the global machine learning market was valued at $8 billion in 2021 and is expected to reach USD 117 billion by 2027, growing at a 39 percent CAGR. Such growth shows the prominent role of ML and, by extension, neural networks in the current tech industry landscape.
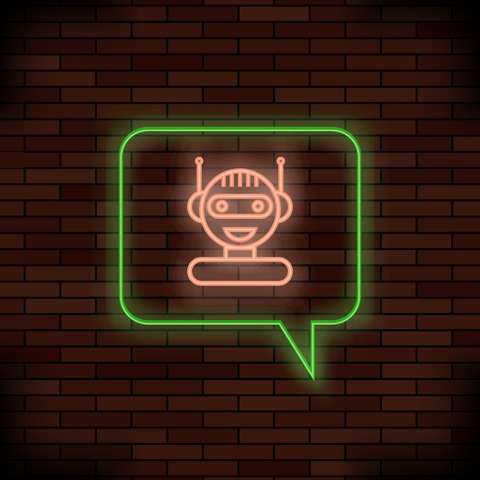